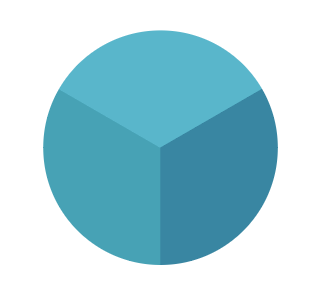
Leaders
Rapid advances in non-invasive neuroimaging methods have revolutionized the possibilities to study changes occurring in living brain across a variety of time-scales ranging from seconds to an entire life span. A large part of these advances can be attributed to the development of dedicated computational algorithms, which are essential in extracting quantitative information from images. The group develops such computational methods to analyze the brain imaging data.
Our current research focus lies in identifying biomarkers of brain disorders from neuroimaging data, which is an exciting and rapidly growing research area at the intersection of machine learning, biomedical engineering and neuroscience. Conventional approaches towards imaging biomarkers reduce the data dimensionality by averaging the image information to one or few variables of a-priori interest – for example, the volume of Hippocampus for Alzheimers diagnosis. However, such methods discard much information present in brain images. Instead, allowing machine learning algorithms to decide what is important and decipher the predictive pattern (sometimes called statistical biomarker) is projected to be beneficial. This leads to challenging and underconstrained machine learning problems where the data dimensionality is larger than the number of samples and advanced computational techniques are required to solve these problems. We develop these techniques and apply to them to large brain image databases to help neuroscientists to find imaging markers to different brain disorders.
Projects
-
NeuroSynth: Simulation of multimodal, longitudinal datasets enabling neurodegeneration tracking
-
Enhancing the innovation potential by advancing the know-how on biomedical image analysis - Kuopio Biomedical Image Analysis Center (KUBIAC) Finished 01.10.2019 - 30.06.2022
-
UEF Brain Research Unit 2.0 Finished 01.03.2023 - 28.02.2025
-
NOVEL MSCA Postdoctoral Programme 01.12.2024 - 30.11.2029
-
Neuro-Innovation 01.05.2021 - 30.04.2026
Cooperation
-
GenomMed Finished 01.04.2018 - 31.12.2022
-
Multiscale Imaging Group 01.09.2015 -
-
Biomedical MRI 01.01.1995 -
-
UEF - Nordic-UK Brain Network (UEF-NBN) 01.01.2022 -
-
NPH and Early AD 01.01.2010 -
-
Clinical Epilepsy Research 01.01.2010 -
-
Neuroinflammation research group 01.01.2017 -
-
Biophysics of Bone and Cartilage (BBC) 01.01.1998 -
-
Brain Research Unit (BRU) - Clinical Trial Unit
-
Molecular Genetics of Alzheimer's Disease - Hiltunen Lab 01.01.2022 -
Keywords
Leaders
Senior Researchers
Post-doctoral Researchers
-
Elaheh Moradi
Postdoctoral ResearcherA.I. Virtanen Institute for Molecular Sciences, Faculty of Health Sciences -
Mastaneh Torkamani Azar
Postdoctoral ResearcherA.I. Virtanen Institute for Molecular Sciences, Faculty of Health Sciences -
Maryam Hadji
Postdoctoral ResearcherA.I. Virtanen Institute for Molecular Sciences, Faculty of Health Sciences -
Mithilesh Prakash
Postdoctoral ResearcherA.I. Virtanen Institute for Molecular Sciences, Faculty of Health Sciences
Doctoral Researchers
-
Ali Farki
Doctoral ResearcherA.I. Virtanen Institute for Molecular Sciences, Faculty of Health Sciences -
Gaurang Sharma
Doctoral ResearcherA.I. Virtanen Institute for Molecular Sciences, Faculty of Health Sciences -
Zewen Zhuo
Doctoral ResearcherA.I. Virtanen Institute for Molecular Sciences, Faculty of Health Sciences
Supporting Staff
Other group members
-
Robert Ciszek Doctoral student
Publications
95 items-
Epilepsy Diagnosis from EEG Signals Using Continuous Wavelet Transform-Based Depthwise Convolutional Neural Network Model
Dişli, Fırat; Gedikpınar, Mehmet; Fırat, Hüseyin; Şengür, Abdulkadir; Güldemir, Hanifi; Koundal, Deepika. 2025. Diagnostics. 15: A1 Journal article (refereed), original research -
A Hybrid End-to-End Spatio-Temporal Attention Neural Network with Graph-Smooth Signals for EEG Emotion Recognition
Sartipi, Shadi; Torkamani-Azar, Mastaneh; Cetin, Mujdat. 2024. IEEE transactions on cognitive and developmental systems. 16: 732-743 A1 Journal article (refereed), original research -
ComBating inter‐site differences in field strength: harmonizing preclinical traumatic brain injury MRI data
Quach, Mara; Ali, Idrish; Shultz, Sandy R; Casillas‐Espinosa, Pablo M; Hudson, Matthew R; Jones, Nigel C; Silva, Juliana C; Yamakawa, Glenn R; Braine, Emma L; Immonen, Riikka; Staba, Richard J; Tohka, Jussi; Harris, Neil G; Gröhn, Olli; O'Brien, Terence J; Wright, David K. 2024. Nmr in biomedicine. 37: A1 Journal article (refereed), original research -
Estimating Dementia Onset: AT(N) Profiles and Predictive Modeling in Mild Cognitive Impairment Patients
Platero, Carlos; Tohka, Jussi; Strange, Bryan. 2024. Current alzheimer research. 2023; 20: 778-790 A1 Journal article (refereed), original research -
Generative Adversarial Networks (GANs) for Medical Image Processing: Recent Advancements
Ali, Mohd; Ali, Mehboob; Hussain, Mubashir; Koundal, Deepika. 2024. Archives of computational methods in engineering. 2025; 32: 1185-1198 A2 Review article, Literature review, Systematic review -
Intelligence and cortical morphometry: caveats in brain-behavior associations
Lewis, John D; Imani, Vandad; Tohka, Jussi. 2024. Brain structure and function. 229: 1417-1432 A1 Journal article (refereed), original research -
Machine learning prediction of future amyloid beta positivity in amyloid-negative individuals
Moradi, Elaheh; Prakash, Mithilesh; Hall, Anette; Solomon, Alina; Strange, Bryan; Tohka, Jussi; Alzheimer’s Disease Neuroimaging Initiative. 2024. Alzheimer's research and therapy. 16: . 46 A1 Journal article (refereed), original research -
Multi-objective genetic algorithm for multi-view feature selection
Imani, Vandad; Sevilla-Salcedo, Carlos; Moradi, Elaheh; Fortino, Vittorio; Tohka, Jussi; for the Alzheimer’s Disease Neuroimaging Initiative. 2024. Applied soft computing. 167: . 112332 A1 Journal article (refereed), original research -
No-Clean-Reference Image Super-Resolution: Application to Electron Microscopy
Khateri, Mohammad; Ghahremani, Morteza; Sierra, Alejandra; Tohka, Jussi. 2024. IEEE Transactions on computational imaging. 10: 1094-1110 A1 Journal article (refereed), original research -
Optimizing Feature Selection for Binary Classification with Noisy Labels: A Genetic Algorithm Approach
Imani, Vandad; Moradi, Elaheh; Sevilla-Salcedo, Carlos; Fortino, Vittorio; Tohka, Jussi. Teoksessa: Daimi, Kevin; Al Sadoon, Abeer(toim.) , 2024. Proceedings of the Second International Conference on Advances in Computing Research (ACR’24). s. 392-403. Springer A4 Conference proceedings