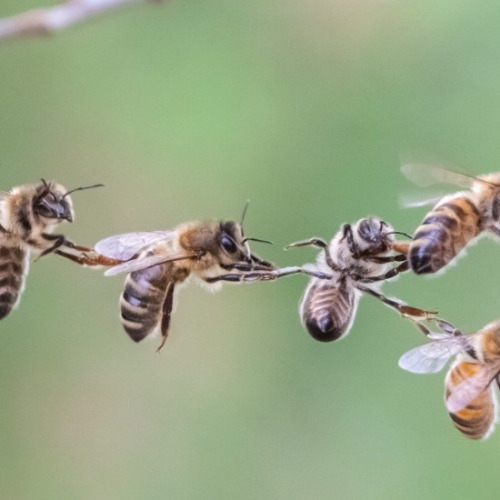
Nature-Inspired Machine Learning
The Nature-Inspired Computing (NIC) methods use the nature as a metaphor, inspiration, and enabler. The typical NIC algorithms include but are not only limited to Neural Networks (NN), Fuzzy Logic (FL), Genetic Algorithms (GA), Tabu Search (TS), Particle Swarm Optimization (PSO), Ant Colony Optimization (ACO), Bacteria Foraging (BF), Differential Evolution (DE), Clonal Selection Algorithm (CSA), Harmony Search (HS), Cultural Algorithms (CA), Simulated Annealing (SA), and Memetic Computing (MC). The Nature-Inspired Machine Learning group focuses on the study of the NIC methods with applications in data mining, optimization, decision making, control, etc. The developed techniques are indeed efficient and effective at manipulating with the uncertainties and imprecision existing in the real-world problems.
Projects:
BioColour (https://biocolour.fi/en/frontpage/)
Recent publications:
[1] W. Yu, J. Fu, Y. Zhao, H. Shi, X. Chen, S. Shen, and X. Z. Gao, “Link prediction in bipartite networks via deep autoencoder-like nonnegative matrix factorization,” Applied Soft Computing, vol. 169, 2025.
[2] X. F. Ji, Y. Zhang, C. He, J. Cheng, D. W. Gong, X. Z. Gao, and Y. Guo, “Surrogate and autoencoder-assisted multitask particle swarm optimization for high-dimensional expensive multimodal problems,” IEEE Transactions on Evolutionary Computation, vol. 28, 2024.
[3] H. Wu, X. Z. Gao, and J.-N. Heng, “Bio-multisensory-inspired gate-attention coordination model for forecasting short-term significant wave height,” Energy, vol. 294, 2024.
[4] Y. Zhang, X. F. Ji, X. Z. Gao, D. W. Gong, and X. Y. Sun, “Objective-constraint mutual-guided surrogate-based particle swarm optimization for expensive constrained multimodal problems,” IEEE Transactions on Evolutionary Computation, vol. 27, 2023.
[5] T. Ye, Z. Zheng, X. Li, Z. Zhao, and X. Z. Gao, “An efficient few-shot object detection method for railway intrusion via fine-tune approach and contrastive learning,” IEEE Transactions on Instrumentation and Measurement, vol. 72, 2023.
Group leader:
Prof. Xiao-Zhi Gao ([email protected])
Group members:
Hatim Arifi
Zhanjun Tan